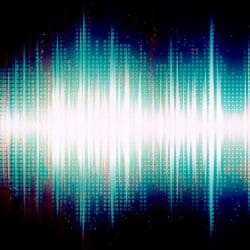
Putting Music Back In Music Discovery: Using Audio Analysis For Better AI
In his Hypebot article about the advent of AI’s use in A&R, Tommaso Rocchi details how the use of Artificial Intelligence now more than ever validates A&R decisions. Predictive modeling is a responsible check-and-balance system to confirm intuitions, but does adopting a numbers-first model in music discovery (i.e examining an artist’s social reach or playlist streams) create more of the same, generic music – and lead to great artists going undiscovered? What if the waves of data overload we receive are in fact crashing over us, rather than allowing us to surf their crest?
By Zach Miller-Frankel, Co-Founder and CEO of Andrson
To improve A.I. in music discovery, we must ask how we can leverage the abundance of data to uncover more artists, qualify their viability, and enhance the discovery process to yield better results. The simple answer? Focus on what matters most: the music.*
Audio analysis remains commercially underutilized and mis-prioritized within the industry. It provides unbiased feedback about the music rather than the artist, and through prioritizing musical data, you can create a model that ultimately increases market size, listening pool, content creation, and profit.
To explore this, we should consider how audio analysis is or can be used today. The diagram below outlines aspects impacting the ecosystem and its major stakeholders: the artist, the industry, and the consumer.
Hundreds of data points within any song can be analyzed. For instance, hook and chorus detection can be isolated. Similarly, melody separation and vocal quality are becoming important analytics. Andrson’s analysis of them allows us to produce sophisticated and better-than-state-of-the-art (and patented) analysis engines.
For the industry, audio analysis provides relevant results that can quickly identify trends, create a more diverse, audio-driven ecosystem, and enable IP protection. We must rely on data in a conformational way, but prioritize the right data at the right time, and for the right purposes. Here’s how the industry can benefit from a music-first approach:
The Labels
Label decisions are governed by nonmusical data, and for the most part that works. Some data analytics tools—Chartmetric, Sodatone, Instrumental—are brilliant for making informed, compelling decisions. But what happens when great artists don’t gain the traction those platforms monitor because they can’t achieve the results necessary to register on them? TikTok is the obvious current answer, but there’s no reliable way for undiscovered artists to breach the data wall.
A&R
For A&R, finding artists based on similarity can equate to faster, greater ROI. Teams can better estimate upfront costs, predict the artists’ trajectory, and pre-define their market. Signing artists earlier allows labels to undercut competition, increases buying power, and creates a pipeline shaped by their own KPIs. This is where TikTok falters—where decision making is based on virality—and audio analysis proves more valuable.
Music Supervisors
Music supervisors are tasked with a challenging job: finding the perfect fit. It’s a Sisyphean task because of the sheer scope; it’s also aggravated by inefficient tools and strict budgets. There is a way to cut through the noise though. Searching by similar artists replaces ‘sorry, we can’t afford Gimme Shelter’ with ‘I know the sound I want! I can’t afford the Stones, but I can get a list of artists that sound similar for much less’. Relying on sound-focused search can provide answers in seconds and greatly reduce spend.
DSPs
Streaming platforms have a unique opportunity to improve their recommender systems. Many DSPs use audio analysis, but their data points tend to be esoteric and they don’t seem to prioritise it in their recommender systems. Rather than recommending music based on sound similarities, they most often rely on user behaviour and geologation; interests tagged by metadata, and it mostly benefits popular musicians. How effective is this for genuine artist discovery? As Cheng et al commented in their study:
“While this method is effective for popular artists with large fanbases, it can present difficulties for recommending novel, lesser known artists due to a relative lack of user preference data.”
We know acoustic similarity is vital to listeners when recommending not just popular artists, but also undiscovered acts, so why isn’t pure sonic analysis used in more effective ways?
Perhaps because DSPs’ goals are to keep users listening longer, so they recommend more popular artists; perhaps partnerships with the major labels also have an impact on the artists being recommended to their end users.
Two key factors to sustain user engagement are optimization and interest. To do this at scale, DSPs need to focus on musical similarity, especially to discover lesser-known acts. It will ultimately lead to a more relevant listening experience.
Rights management and artistic development
Let’s look at two more applications: rights management and artistic development. About $110 million is spent on infringement litigation and MBW estimates tens of millions of pounds is lost in royalties annually. And while the royalty “storm” that the BBC reported on largely relates to Covid, if PRS processed 13 trillion “performances” last year, consider PROs worldwide. How much revenue fell through the cracks because of missing metadata; incorrect or forgotten ISRC codes; unreliable fingerprinting, etc.?
Relying more on AI—song-to-song analysis to compare structures like tune and arrangement—evolves forensic musicology. It can, as lawyer Peter Mason confirms in Raconteur’s article, “analyse two songs and say [they’re] similar in instrumentation sound, but also [this or that feature] is not relevant, so you might be able to cut out elements which shouldn’t be protected as part of the composition.”
So whether you’re arguing if the lines are truly blurred between Marvin Gaye and Robin Thicke, debating if the building blocks of music can be owned to the point of IP theft, or wondering if your PRO statement is 100% accurate, a scientific approach can help.
Artists
Audio analysis can be invaluable for artists. To be clear, we’re not advocating its use to change an artist’s music. But getting unbiased feedback can frame a musician’s point of view in a way perhaps their own bias cannot. It may inspire innovation and stylistic evolution. It can help them hone their market, focus their branding, and create more music. For independent artists this means easier market traction; for artists who want a major agent or label, it can encourage commerciality faster and more reliably than numbers alone.
In summary…
Ultimately this is what consumers want: artists whose music they can rally behind. Playlists introducing them to new musicians similar to acts they love and the stickiness of believing they heard it first. For artists that equates to greater loyalty. For the industry it leads to longer platform engagement, higher streaming numbers, and more tickets sold. And all that, just from the music.
But when data is the tool and traction is proof, it becomes the responsibility of decision makers to temper numbers with what still remains the most powerful tool in the industry: the human ear. So, does adopting a numbers-first discovery method breed more of the same, generic music? It does. And what if in fact we are not utilizing the data in the right way? We aren’t. Because of that, great music is getting lost. The next question we must ask ourselves is: do we care enough to change it?
Very interesting article! Thank you for your time!